Disordered Systems at (Net)work
"I’m broadly interested in disordered matter… liquids, glasses, disordered systems" says Professor Mark Wilson and from perusing just a few of the recent publications from the group it is clear there exists a wide range of diverse systems covered under this umbrella that encompass multiple facets of chemistry including inorganic, physical, and biological. A recent theme in the group has been that of the construction and optimisation of 2D planar networks, which are representations of systems using connected 2D polygons. An example of a very large and famous network of this kind is the Giant’s Causeway, a collection of naturally formed basalt columns in Northern Ireland. What makes this site special are the shapes in which the formation has developed – if one could fly over the region of tall columns and take a bird’s eye view, what would be seen is a series of connected polygons, such as hexagons and pentagons, which form a large 2D network. These sorts of networks can occur on the very large and very small scale, and the work such as that carried out in the Wilson group can help to predict the structures of these systems using computational techniques.
An example small system where this occurs is in bilayers of silicon dioxide and recently the Wilson group published a method which could predict the development of these layers based on a set of rules which allowed a seed to grow into a larger network (Morley & Wilson, 2019). SiO2 has topological features such as ring sizes (tetrahedra can join together to form a ring, but the number of members of the ring can vary) and ring distribution (a measure of the positioning of different ring sizes in the network and which sizes are likely to appear adjacent to each other). The reported work detailed a method for following these empirical laws and, using this information, then predicted the growth of bilayers depending on different temperatures.
The Wilson group have looked at a large number of Si systems, such as that described above, but recently the interest in 2D networks has also moved out of the inorganic realm and into the biological one with Mark explaining that when you can understand well the behaviour of silicon, moving into a biological system is a sensible move: “[SiO2] networks are very rigid, [biological] networks are much looser so it’s a natural progression”. A recent paper from the group (Bailey et al, 2020) looked at further developing an existing algorithm to be applicable to biological systems such as cell membranes.
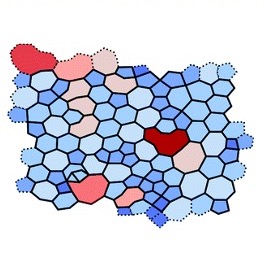
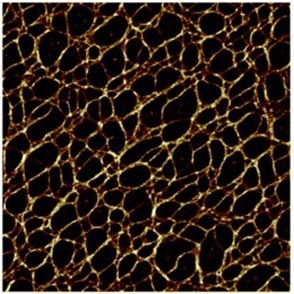
Figures. An example of simulation results of a planar network (left) compared to experimental results (right) for collagen fibres. Images from Bailey et al. (2020) and Wang et al. (2017).
In this paper, a network of tessellated hexagons (think honeycomb structure) was chosen as the starting point, and a bond-switching algorithm applied that altered the network step by step (and consequently shape by shape) into a new network. This bond switch, as suggested by the name, moves one bond between two vertices to another position within the network, so instead of a bond being between vertices A and B, it is now between A and C. As a result, two polygons can change in size and shape, in terms of number of edges and vertices, and the new network is accepted or rejected based on, in this case, the change in potential energy after optimisation. Generally speaking, the new network will be accepted as a successful modification if the ‘new’ potential energy is more favourable than the previous or ‘old’ one. These networks can then be compared to experimental images to measure how successful the new algorithm is at predicting network shape and structure. This method has interesting future implications for biological materials such as collagen, which is very difficult to experimentally image, so being able to accurately predict its structure is important as it could provide more information about this network and how it changes over time.
Mark also recently contributed to a Nature paper (Deringer et al., 2021) which developed a new machine learning method that accurately predicted the phase transition structure of amorphous silicon and included the exciting discovery of a very high density amorphous (VHDA) phase. This work, led by Volker Deringer, was particularly impressive since a) such predictions and discoveries had not been achieved before now through either experiment or simulation, and b) this method can be applied to other systems, not just silicon ones. When asked how he got involved in this project, Mark said, “Volker had been doing work [on this system] for a while… [and had] known or realised it was an interesting system”, and with Mark’s knowledge of Si he said it was a “nice, natural, collaboration”.
Reported on here are only a couple of examples of the works from the Wilson group but it is clear that their interests are diverse and their interest in solving problems covers a range of disciplines so it will be fascinating to see where this work takes them next!
Article by Hannah Fowler
Many thanks to Matthew Bailey and Mark Wilson for their help with this article.
Read the full papers:
D. O. Morley & M. Wilson, Molecular Physics, 2019, 117, 3148
M. H. J. Bailey et al., RSC Adv., 2020, 10, 38275
V. L. Deringer et al., Nature, 2021, 589, 59
Wang et al., J. Food. Qual., 2017, 2017, 1-10