Machine-learning models to create and explain atomic disorder
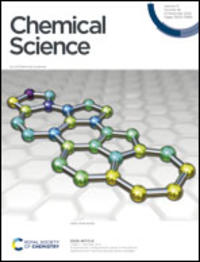
Research from the Department of Chemistry is featured on the cover of this week’s issue of Chemical Science. The study by DPhil student Zakariya El-Machachi, from the Deringer research group and the EPSRC TMCS Centre for Doctoral Training, provides new insight into the nature of disordered matter through atomic-scale machine-learning methods.
Most materials have ordered structures, yet those that lack order are often of particular interest. Disordered materials are abundant and have interesting properties: for example, solar cells can be made from amorphous (non-crystalline) silicon, and the glass found in windows is mainly a disordered three-dimensional network of silicon and oxygen atoms.
Disorder is also relevant in two-dimensional systems. Taking graphene (a single layer of graphite) as the most famous 2D material, atoms form an ordered honeycomb structure, consisting entirely of six-membered rings. In their new study, the Oxford team created structural models of disordered graphene and linked the degree of disorder with the stability of these structures. Such disorder, with five-, six-, and seven-membered rings of atoms, is shown in the cover art and in Figure 1.
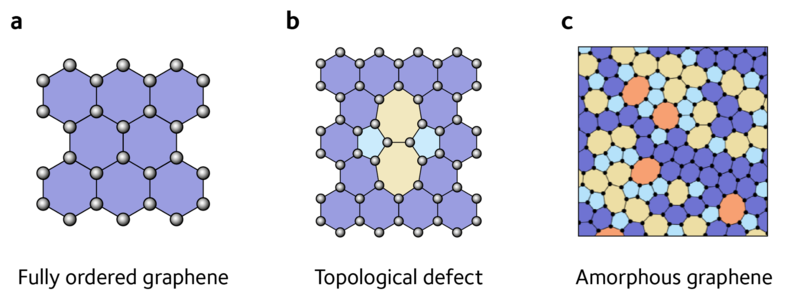
From fully ordered graphene, consisting entirely of six-membered rings, to a single topological defect, and onwards to disordered (amorphous) graphene.
Working out the energetic stability of molecules and solids is not a trivial task, and accurate predictions require computationally expensive quantum-mechanical methods such as density-functional theory (DFT). With this method, system sizes are limited to a few hundred atoms and the simulations require long runtimes, even on supercomputers.
Machine learning (ML) methods can help to overcome this issue. Machine-learned energy and force models can be “trained” on DFT data to achieve a similar accuracy for a small fraction of the cost. This unlocks the ability to model much larger systems for longer, which is key for generating disordered structures systematically.
To create such structures for amorphous graphene, the authors combined their ML model with a bond-switching algorithm, modifying the network topology systematically until the energy of the network converged. A topological constraint was set to ensure each atom must be three-fold coordinated (otherwise the move was rejected). The approach then evaluated the energy of the structure. One of the key assumptions of the ML model used here is that the total energy can be decomposed into sums of local machine-learned atomic energies. These ML atomic energies were used to decide if a move was accepted or not, and thereby to steer the structural search.
With the new structural models in hand, the authors went on to characterise them in more depth. They found that the ML atomic energies provide a characteristic fingerprint for disorder in the structures. A highly disordered structure gave a single peak, whereas structures with more order gave two peaks (see Figure 2). This finding was important as it suggests a new way of characterising disorder in materials.
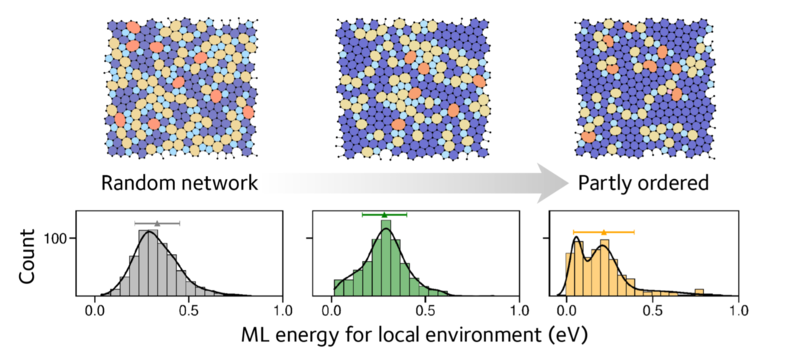
Machine-learned local energies provide fingerprints of disordered structures, from a random network of atoms (left) to a partly ordered, paracrystalline structure that was described recently (right). Both figures are adapted from the paper (see link below), where more details may be found.
First author Zakariya El-Machachi says:
Understanding disordered networks is one of the most fundamental problems in structural chemistry. It is also one of the oldest, dating back nearly 100 years, yet little is known about the atomic arrangement of these networks. We turn to machine-learning models to gain new insight into the apparent medium-range ordering in disordered graphene to help us understand the intricate relationship between energy and structure.
Professor Volker Deringer, senior author of the study, leads a research group in the Inorganic Chemistry Laboratory. His team is focused on combining novel machine-learning techniques with research questions in structural chemistry. To understand the details of the connections between atoms (the topology), the authors collaborated with Professor Mark Wilson in the Physical and Theoretical Chemistry Laboratory who is an expert on describing disordered networks.
Zak’s studies are funded through an EPSRC TMCS CDT studentship (https://www.tmcs.ac.uk/).
You can read more about the study in Chemical Science via Open Access here.